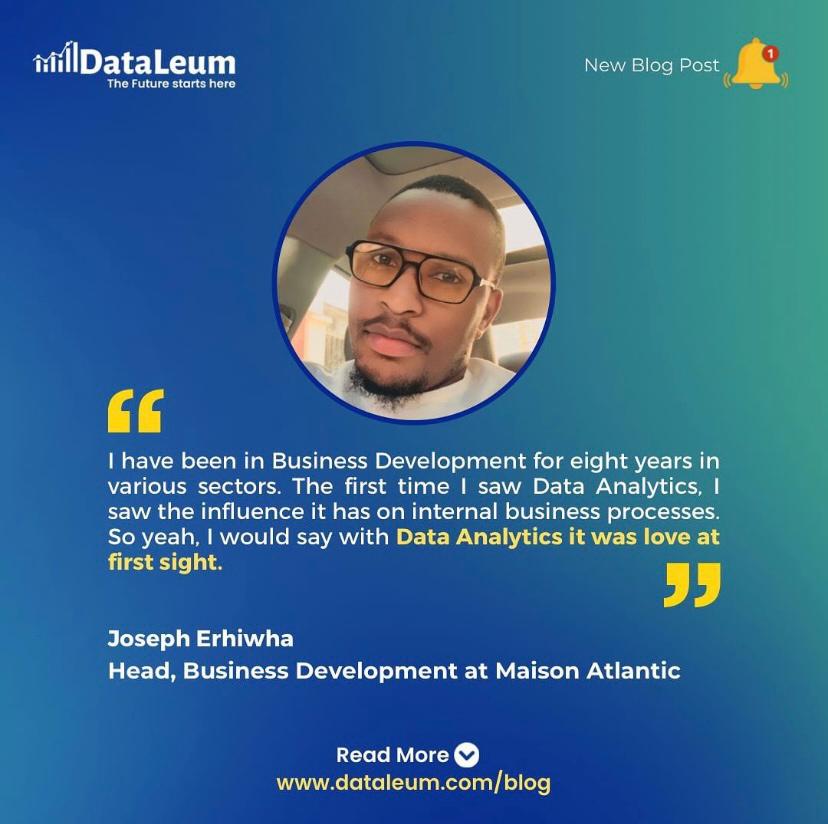
Discovering a passion for data analytics isn’t just a career choice; it’s a love story in itself. In our conversation with Joseph Eriwha, Head of Business Development at Maison Atlantic, we explore how he found himself captivated by the power of data analytics. His story of how data analytics became a vital tool in reviving businesses is a tale worth sharing.
ABOUT JOSEPH ERHIWHA
Holding a bachelor’s degree from Middlesex University, majoring in Information Technology and Business Management Systems, I would classify myself as a seasoned business development expert with a wealth of experience in converting strategic plans into achievable business goals. In over 8 in business development, I have explored various industries and sub-sectors in the technology, power, logistics and hospitality space.
Connect with Joseph Erihwa on LinkedIn.
Was it love at first sight with Data Analytics?
For short, the answer is yes. So I’ve been in business development for eight years in various sectors from financial technology, health technology, logistics technology, information technology and the like.
And the first time I saw Data Analytics, I saw the influence it has on internal business processes. It has a lot of focus on internal business processes, more than the conventional tools that we use as business development officers which include SWOT analysis, Porter 5 forces, PESTEL and the like. It takes into account external factors that we an organisation do not have control over such as your competitors, and force measure. So yeah, I would really say with Data Analytics it was love at first sight.
What sparked your interest in Data Analytics initially?
So I used to be the sales manager for what I will call the biggest telemedicine platform in Nigeria and we saw that we were not really making a lot of growth in terms of customer base. So we had to crunch our numbers to also see the types of people that we have (types of enrollees) on our platform. We saw that the majority of them were working class, and we were able to switch our business model because we utilised Data Analytics.
We were able to switch our business model from B to C to B to B, and easily forecast our financial numbers, also the enrolees base we plan to have within the year, which was easier to do with B2B. So that streamlined our sales activities to achieve individual organizational goals.
Can you share a memorable project or experience that solidified your passion for Data Analytics?
Yes, let me use my most recent place of work, somewhere I currently consult for. It’s in the logistics technology sector and I’ve been able to utilise Data to understand our high-traffic areas, high-traffic customers, the form of demographic and also the psychographic data on why they purchase our products. That way we’ve been able to push our energy to areas that we have a lot of others coming from.
So we are going to have better customer service in terms of speed, pricing and pick up time. It has affected affected our business positively. So instead of just using our numbers or our efforts to capture the whole of Lagos, we try to streamline it to various geographic locations.
In our case, we are using local governments as a basis to look at how to grow our numbers and that is working for us instead of just having scattered sales activities around Lagos.
How has your love for Data Analytics influenced your career and personal growth?
Well, to be honest, I will say it has affected me. In the past, in some of my past activities, we’ve utilised the services of what we call data analysts consultants, people who are not under the payroll but are at our beck and call, based on request.
Now having that skill at hand gives me an edge in the market because most business development officers I know have no knowledge about data. So it gives me an edge because of that skill set. Being able to analyse businesses, the strengths and weaknesses of businesses based on the data they’ve gathered. So informed decisions are made better.
What is your favourite thing about being an Analyst?
I would say it’s something people shy away from, but data cleaning is very important. From my personal experience, just to give you a rough estimate might not be the exact numbers, but roughly about 90% of the time I spend with data analysis is on data cleaning.
You have to have clean data to have accurate results. So spending a lot of time on data cleaning, it’s it’s I think has been my core strength. It’s something I’m still developing because there’s room for growth, but it’s my core strength currently as a Data Analyst. Utilising Excel and PowerBi, I’m currently working on that for SQL and it’s going pretty great so yeah!
We hope you enjoyed reading this article as much as we enjoyed writing it. Our discussion was truly enlightening, emphasizing the incredible potential of data. It’s amazing to see how data can change lives and revolutionize business practices.
If you’re eager to share your own experiences with data analytics, send us a message! We’d love to hear from you.